Part 6 of our ABCs of AI Ethics Series. Read our previous article on Excellence in AI.
As an AI ethics practitioner, I often encounter a moment of revelation when working with organizations. It typically comes after they proudly show me their AI system’s perfect fairness metrics. Last week, it happened again during a consultation with a financial services firm.
“Look,” their CTO said, displaying a dashboard of impeccable equality metrics across all demographics. “Our AI treats everyone exactly the same.”
Then, we examined their weekend data.
The system was 40% less likely to approve transactions on weekends – a subtle but significant bias against small business owners, gig workers, and anyone operating outside traditional banking hours. In pursuing perfect statistical equality, they had inadvertently encoded socioeconomic bias into their system.
The Hidden Complexity of AI Fairness
After analyzing hundreds of AI systems throughout 2024, I’ve discovered an uncomfortable truth: the most sophisticated approaches to fairness often create the most insidious biases. Consider these statistics:
– 72% of enterprise decisions now involve AI
– Organizations lose $3.2M on average from fairness incidents
– 89% of “debiased” systems still show significant bias when tested thoroughly
But here’s what rarely makes it into the white papers and technical documentation: adding more diverse data often makes bias worse. Without understanding systemic biases, you’re just reinforcing them at a larger scale.
Beyond Surface-Level Fairness
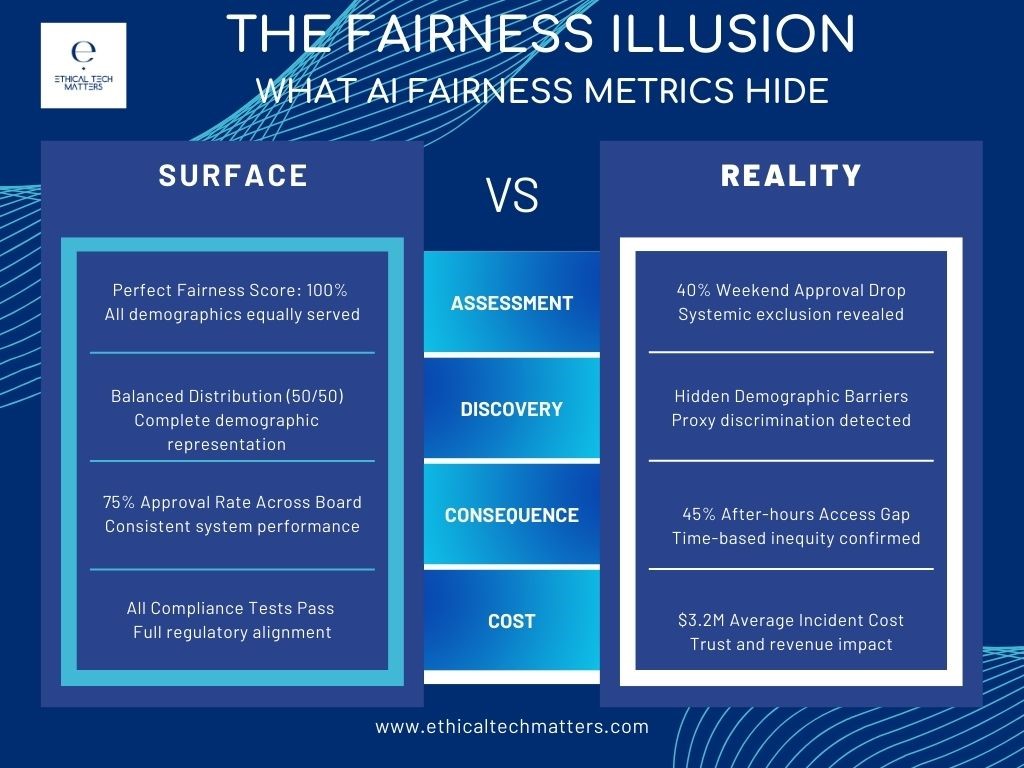
Through implementing fairness frameworks across various organizations, I’ve identified five critical dimensions that every leader must consider:
1. Multiple Perspectives Matter: A healthcare provider’s AI showed no demographic bias in its initial testing. Yet when we examined usage patterns, we discovered that its system was making dramatically different recommendations for night shift workers—not because of explicit bias but because the training data came primarily from daytime patients.
2. Context is Critical: A retail AI that scored perfectly on standard fairness metrics was quietly reshaping community access to essential services. By optimizing for overall efficiency, it had created “AI deserts” in certain neighborhoods, demonstrating how seemingly fair systems can amplify existing social disparities.
3. Impact Over Intent: When a recruitment AI was found to be biasing against candidates over 40, the solution wasn’t in the algorithm – it required rethinking how we measure career success. Technical fixes alone couldn’t address the underlying assumptions about what makes a “good” candidate.
4. Continuous Monitoring is Essential: Your AI system is never “done” being fair. As society evolves, so do biases. A system that’s fair today might not be fair tomorrow, especially as usage patterns and social norms shift.
5. Solutions Must Be Holistic: Technical adjustments matter, but lasting solutions require a broader view. When a financial AI showed bias against women entrepreneurs, the fix involved reconsidering fundamental assumptions about business hours, stability metrics, and success indicators.
Lessons From the Field
After a decade in AI ethics, here are the uncomfortable truths I’ve learned:
1. Perfect metrics usually hide deeper problems
2. The most dangerous biases are often invisible in standard testing
3. User complaints frequently reveal systemic issues
4. Quick fixes typically create bigger problems
5. Real fairness requires continuous attention
Looking Ahead
As we navigate 2025, the stakes for AI fairness have never been higher. Organizations that master fairness won’t just meet compliance requirements – they’ll build the trust necessary for successful AI adoption and innovation.
The future of AI is fair, but only if we make it so. This requires moving beyond simplistic metrics to understand the full complexity of fairness in AI systems. It demands rigorous testing, continuous monitoring, and most importantly, a commitment to seeing beyond surface-level equality to achieve genuine equity.
Take Action
Has this article made you think differently about fairness in your AI systems? Share your experiences and challenges in the comments below. Your insights might help others identify hidden biases in their own systems.
Next in our series: G is for Governance – Understanding the Framework for Responsible AI
—
About the Author: Drawing from extensive experience helping organizations identify and fix AI bias, I focus on building practical frameworks for ethical AI implementation. Connect with me to learn how we can help your organization build truly fair AI systems.*
#AIEthics #FairAI #ResponsibleAI #EthicalTech #ABCsofAIEthics